Unlocking the Power of Image Labeling Tools for Object Detection
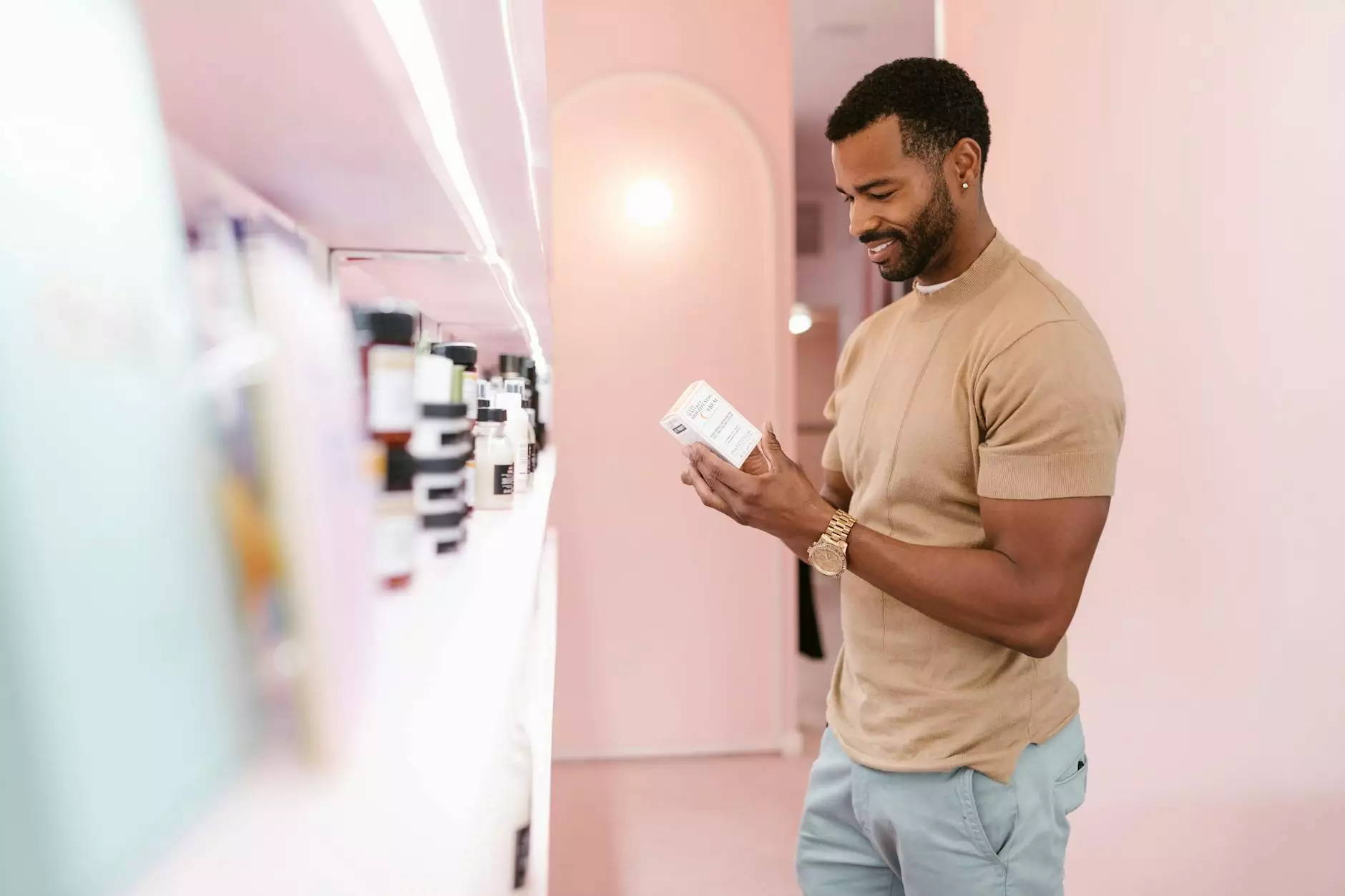
The Importance of Image Labeling Tools in Data Annotation
In today's rapidly evolving technological landscape, image labeling tools for object detection have emerged as pivotal components in training powerful AI models. These tools facilitate the process of tagging images with pertinent information, enabling machines to recognize and classify objects within various visual contexts. Whether used in autonomous vehicles, facial recognition systems, or retail analytics, effective image labeling directly influences the accuracy and efficiency of object detection systems.
What is Image Labeling?
Image labeling, also referred to as data annotation or tagging, involves assigning labels to images or portions of images that signify the objects they contain. This process is essential for supervised learning in machine learning and deep learning algorithms. The image labeling tool for object detection automates and streamlines this vital process, allowing enterprises to create high-quality labeled datasets efficiently.
Why Choose KeyLabs.ai for Image Labeling?
KeyLabs.ai specializes in offering top-notch data annotation tools and an all-encompassing data annotation platform. Here are several reasons why KeyLabs.ai stands out in the realm of image labeling:
- Precision and Accuracy: The platform ensures high-quality labels with minimal error rates, providing results you can trust.
- User-Friendly Interface: KeyLabs.ai offers an intuitive design that makes the labeling process accessible to users of varying technical expertise.
- Scalability: The platform can handle projects of any scale, from small datasets to massive collections of images.
- Collaboration Tools: Teams can work together seamlessly, sharing insights and annotations in a streamlined environment.
- Fast Turnaround Times: High-efficiency workflows ensure that your data is labeled quickly, helping you maintain momentum in your projects.
Understanding Object Detection and Its Applications
At its core, object detection refers to the process of identifying and locating objects within an image. This involves not only determining the presence of objects but also pinpointing their locations through bounding boxes or segmentation masks. The applications of object detection are vast and continually expanding:
Key Applications of Object Detection
- Autonomous Vehicles: Vehicle navigation systems leverage object detection to identify pedestrians, cyclists, and other vehicles on the road.
- Healthcare: Advanced imaging tools utilize object detection for identifying anomalies in medical scans.
- Retail: Customer behavior analysis and inventory management can benefit from observing shopper actions through object detection.
- Security: Surveillance systems use object detection for real-time monitoring of environments.
- Manufacturing: Quality control processes are enhanced by analyzing product defects with object detection mechanisms.
The Science Behind Image Labeling Tools
The effectiveness of image labeling tools for object detection hinges on several key components:
1. Annotation Types
Different types of annotations serve various purposes in object detection. Commonly used annotation types include:
- Bounding Boxes: Rectangles drawn around objects to define their locations.
- Polygonal Segmentation: Precise outline of object shapes for detailed context.
- Keypoints: Specific points on objects that denote critical features (e.g., joints in human figures).
2. Labeling Techniques
There are several techniques for labeling images effectively:
- Manual Labeling: Involves human annotators to apply labels, which can be time-consuming but very accurate.
- Automated Labeling: Uses machine learning algorithms to predict labels, significantly speeding up the process.
- Active Learning: A hybrid approach that combines human oversight with automated processes for high accuracy.
Choosing the Right Image Labeling Tool
When selecting an image labeling tool, it's vital to consider several factors:
1. Budget Constraints
Analyze the cost-effectiveness of the platform without compromising on quality.
2. Volume of Data
Evaluate if the tool can handle the volume of images you need to annotate without delay.
3. Support for Multiple Formats
Ensure the platform supports diverse image formats and integrates easily with your existing workflows.
4. Quality Assurance Features
A good image labeling tool should have quality checks to maintain high standards in data quality.
5. Customer Support
Reliable customer support can ease the transition and handling of technical issues as they arise.
Real-World Impact of Effective Image Labeling
Implementing strong image labeling practices has profound implications across various industries. Here are a few case studies demonstrating the effectiveness of image labeling tools for object detection:
Case Study 1: Enhancing Healthcare Diagnostics
In a recent project, a healthcare technology firm utilized an image labeling tool to analyze x-ray images for anomaly detection. By implementing accurate data annotation, the company improved early detection rates by 25%, leading to timely interventions for patients.
Case Study 2: Transforming Retail Experiences
A leading retail organization harnessed image labeling to analyze customer behavior patterns in stores. By accurately labeling shopper interactions, the company gained actionable insights, optimizing store layouts and increasing sales conversions by 15%.
Future Trends in Image Labeling and Object Detection
As technology continues to advance, the field of image labeling will evolve, leading to several emerging trends:
1. Integration of AI and Automation
The integration of artificial intelligence will streamline image labeling processes, reducing the need for manual input while enhancing accuracy.
2. Real-time Annotation
Real-time image labeling capabilities will allow for immediate feedback and rapid deployment in scenarios requiring quick decisions, such as surveillance.
3. Ethical Considerations
As the reliance on data grows, so do the ethical implications surrounding data privacy and labeling bias. Organizations must strive for transparency and fairness in their labeling processes.
Conclusion
In summary, the effectiveness of any image labeling tool for object detection lies in its ability to produce high-quality datasets that empower machine learning models. KeyLabs.ai stands at the forefront of this critical endeavor, delivering top-tier data annotation tools and a comprehensive data annotation platform that meets a variety of industry needs. As the landscape of AI evolves, investing in robust image labeling solutions will be paramount for organizations aiming to achieve competitive advantages and drive innovation.